Ahmad Esmaeili
School of Computing, Wichita State University.
JB 221, 1845 Fairmount St.
Wichita, KS 67260
I am an Assistant Professor of Computer Science in the School of Computing at Wichita State University, where I lead the Multi-Agent Systems Research Lab. My work focuses on distributed artificial intelligence, exploring multi-agent systems, machine learning, and collaborative approaches to design intelligent, autonomous technologies for next-generation cyber-physical applications.
Before joining WSU, I earned my Ph.D. and served as a graduate instructor in the Department of Computer and Information Technology at Purdue University, West Lafayette.
I have multiple Ph.D. openings in my research lab for motivated students with a strong background in machine learning, mathematics, and programming. If you are interested in working on cutting-edge research in multi-agent systems and distributed artificial intelligence, please fill out this form to express your interest. I will review submissions and reach out to strong candidates for further discussion.
For efficiency, please use the form instead of emailing me directly, unless you encounter access issues.
news
Nov 18, 2024 | Our paper on Hybrid Algorithm Selection and Parameter Tuning has been accepted in the ACM Transactions on Internet Technology. |
---|---|
Sep 4, 2024 | Our paper on Multi-section Hierarchical Deep Neural Network has been accepted in IEEE Access. |
Aug 1, 2024 | I joined the School of Computing at Wichita State University. |
Apr 1, 2024 | Our paper on Fuzzy Q-Table RL has been accepted in IEEE CoDIT 2024. |
Dec 20, 2023 | Our paper on Holonic Learning has been accepted in the main track of AAMAS 24. |
Nov 9, 2023 | Our paper on Cross-individual Huma Activity Recognition paper accepted in the IEEE Robotoc Computing. |
teaching
CS560: Design and Analysis of Algorithms (Fall 2024, Spring 2025) – WSU
CS797O: Neural Networks and Deep Learning (Fall 2024) – WSU
CNIT175: Visual Programming (Fall 2020 – Summer 2024) – Purdue
Introduction to Artificial Intelligence (Spring 2020) – KSW-Purdue
Introduction to Machine Learning and Deep Learning (Summer 2019) – KSW-Purdue
selected publications
For the most up-to-date list of publications, please visit the Google Scholar page.
- ACM TOITHybrid Algorithm Selection and Hyperparameter Tuning on Distributed Machine Learning Resources: A Hierarchical Agent-based ApproachACM Transactions on Internet Technology 2024
- IEEE AccessA Multi-Section Hierarchical Deep Neural Network Model for Time Series Classification: Applied To Wearable Sensor-Based Human Activity RecognitionIEEE Access 2024
- AAMASHolonic Learning: A Flexible Agent-based Distributed Machine Learning FrameworkIn Proceedings of the 23rd International Conference on Autonomous Agents and Multiagent Systems 2024
- SystemsAgent-based Collaborative Random Search for Hyper-parameter Tuning and Global Function OptimizationSystems 2023
- ACM TAASHAMLET: A Hierarchical Agent-based Machine Learning PlatformACM Transactions on Autonomous and Adaptive Systems, 2022
recent projects
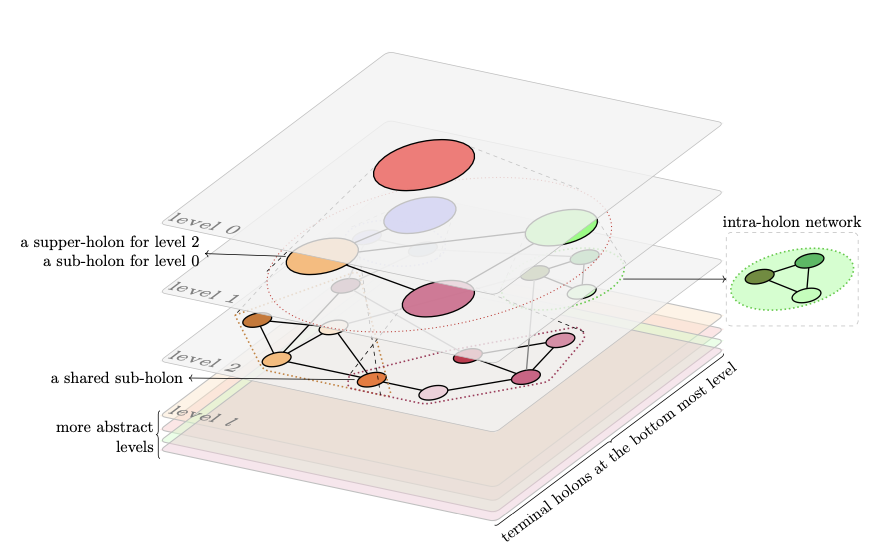
Holonic Learning
A research on designing a collaborative and privacy-focused framework for training deep learning models, leveraging structured self-similar hierarchies and individual model aggregation within holons to address scalability, resource distribution, and privacy concerns in the context of increasingly distributed machine learning paradigms.
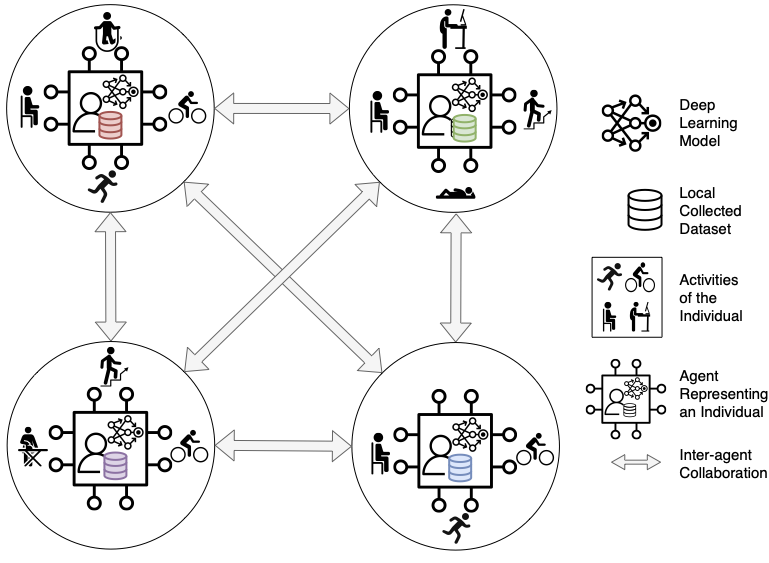
Distributed Cross-Individual Human Activity Recognition
A research on a collaborative distributed learning approach rooted in multi-agent principles for decentralized Human Activity Recognition, leveraging wearable sensor technologies to uphold privacy, eliminate external server dependencies, and demonstrate superior effectiveness in local and global generalization.
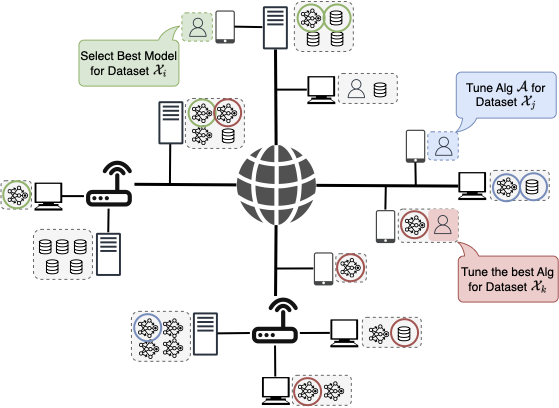
Agent-based Distributed ML Algorithm Selection and Tuning
A research on developing a fully automated and collaborative agent-based mechanism for ML algorithm selection and hyperparameter tuning, utilizing resources organized distributedly by a hierarchical machine-learning platform.
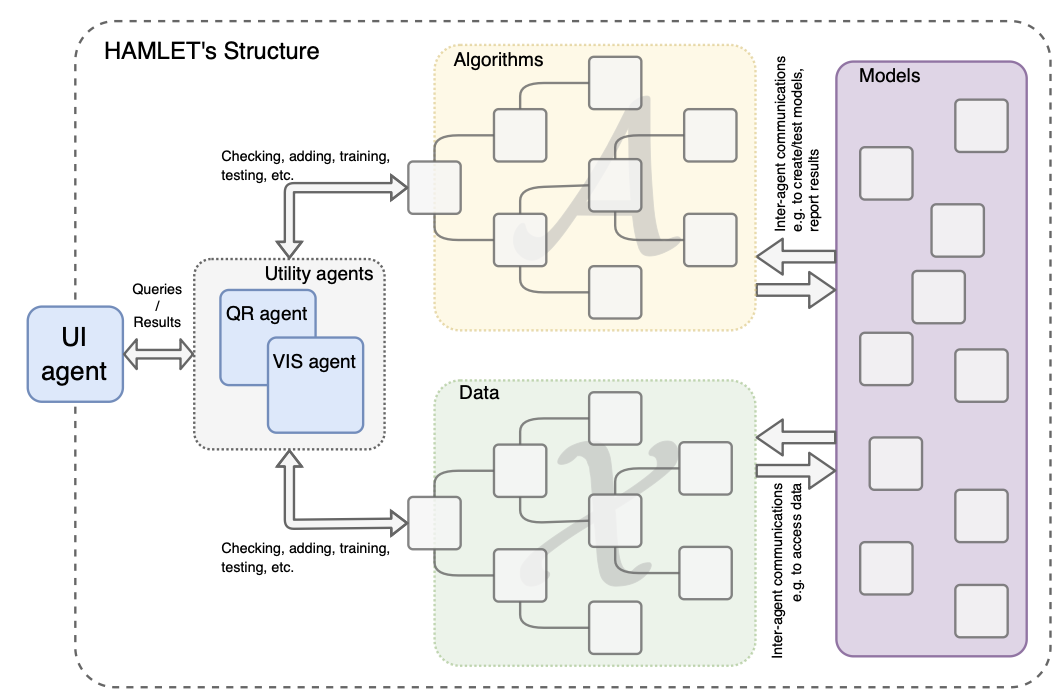
Agent-based Modelling of Distributed Machine Learning Systems
A research on building a hybrid machine learning platform that leverages Multi-Agent Systems to autonomously organize and democratize geographically distributed ML resources and offers analytical capabilities for robust research assessment across various algorithms and datasets.